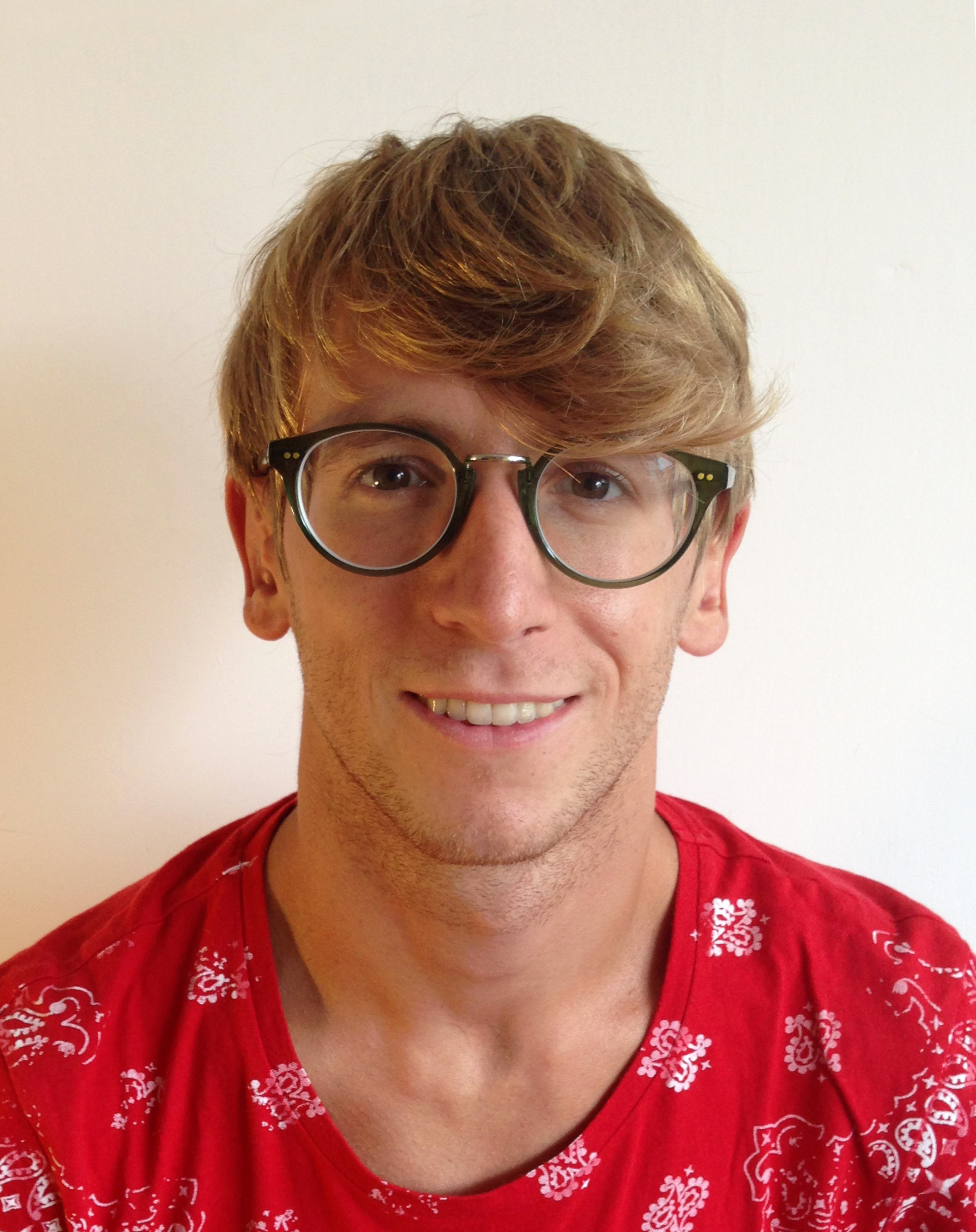
I am Stefano Sarao Mannelli, a Senior Reseach Fellow working with Andrew Saxe at Gatsby and SWC (University College London). Prior to my current position, I held a postdoctoral position at the University of Oxford (still with Andrew Saxe) and obtained a Ph.D. in Physics applied to Machine Learning at the University of Paris-Saclay supervised by Lenka Zdeborova. My research focuses on analysing machine learning problems using a model-based approach, where the complexity of the problem is reduced to obtain a parsimonious solvable model that still captures the phenomenon of interest. In my previous works, I applied several variations of this approach to study problems in learning, such as transfer learning, continual learning, and curriculum learning.
Contacts and information
Publications
Accepted to ICLR 2025
CogSci 2024 (Oral)
Accepted to NeurIPS 2024
ICML 2024
ICML 2024
Accepted to PRX
NeurIPS 2022
ICML 2022
Machine Learning: Science and Technology
NeurIPS 2021
Proceedings of the National Academy of Sciences
NeurIPS 2020
NeurIPS 2020
Journal of Statistical Mechanics: Theory and Experiment
Physical Review X
NeurIPS 2019 (Spotlight)
ICML 2019