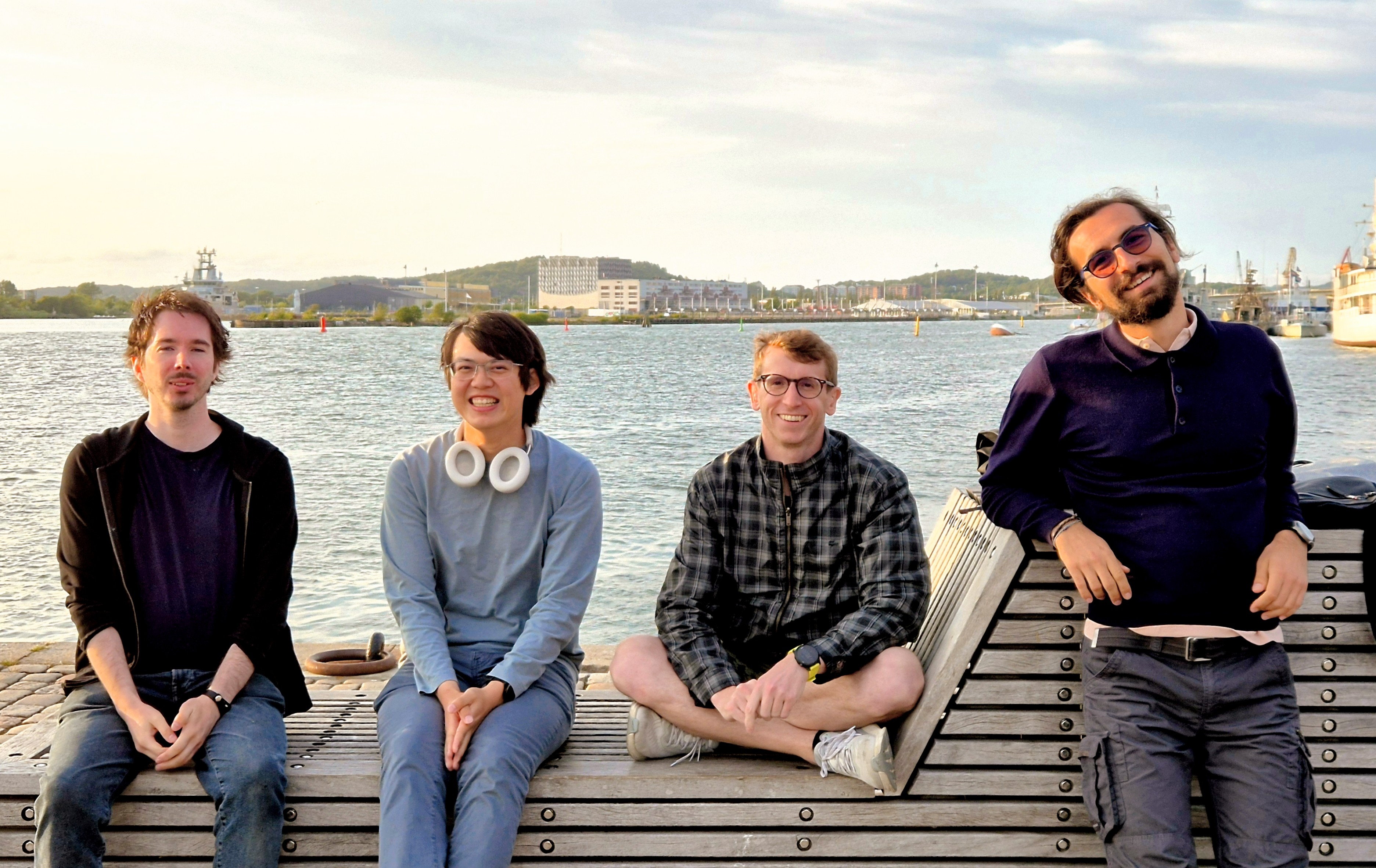
Latest News
You can find all the news here.
Recent publications
- The sequencing of training trials can significantly influence learning outcomes in humans and neural networks. However, studies comparing the effects of training curricula between the two have typically focused on the acquisition of multiple tasks. Here, we investigate curriculum learning in a single perceptual decision-making task, examining whether the behavior of a parsimonious network trained on different curricula would be replicated in human participants. Our results show that progressively increasing task difficulty during training facilitates learning compared to training at a fixed level of difficulty or at random. Furthermore, a sequences designed to hamper learning in a parsimonious neural network... [Read Article]
A Theory of Initialisation's Impact on Specialisation
Devon Jarvis, Sebastian Lee, Clémentine Carla Juliette Dominé, Andrew M Saxe, Stefano Sarao MannelliPrior work has demonstrated a consistent tendency in neural networks engaged in continual learning tasks, wherein intermediate task similarity results in the highest levels of catastrophic interference. This phenomenon is attributed to the network's tendency to reuse learned features across tasks. However, this explanation heavily relies on the premise that neuron specialisation occurs, i.e. the emergence of localised representations. Our investigation challenges the validity of this assumption. Using theoretical frameworks for the analysis of neural networks, we show a strong dependence of specialisation on the initial condition. More precisely, we show that weight imbalance and high weight entropy can favour... [Read Article]Optimal Protocols for Continual Learning via Statistical Physics and Control Theory
Francesco Mori, Stefano Sarao Mannelli, Francesca MignaccoArtificial neural networks often struggle with catastrophic forgetting when learning multiple tasks sequentially, as training on new tasks degrades the performance on previously learned ones. Recent theoretical work has addressed this issue by analysing learning curves in synthetic frameworks under predefined training protocols. However, these protocols relied on heuristics and lacked a solid theoretical foundation assessing their optimality. In this paper, we fill this gap combining exact equations for training dynamics, derived using statistical physics techniques, with optimal control methods. We apply this approach to teacher-student models for continual learning and multi-task problems, obtaining a theory for task-selection protocols maximising... [Read Article]A meta-learning framework for rationalizing cognitive fatigue in neural systems
Yujun Li, Rodrigo Carrasco-Davis, Younes Strittmatter, Stefano Sarao Mannelli, Sebastian MusslickThe ability to exert cognitive control is central to human brain function, facilitating goal-directed task performance. However, humans exhibit limitations in the duration over which they can exert cognitive control -a phenomenon referred to as cognitive fatigue. This study explores a computational rationale for cognitive fatigue in continual learning scenarios: cognitive fatigue serves to limit the extended performance of one task to avoid the forgetting of previously learned tasks. Our study employs a meta-learning framework, wherein cognitive control is optimally allocated to balance immediate task performance with forgetting of other tasks. We demonstrate that this model replicates common patterns of... [Read Article]Bias in Motion: Theoretical Insights into the Dynamics of Bias in SGD Training
Anchit Jain, Rozhin Nobahari, Aristide Baratin, Stefano Sarao MannelliMachine learning systems often acquire biases by leveraging undesired features in the data, impacting accuracy variably across different sub-populations. Current understanding of bias formation mostly focuses on the initial and final stages of learning, leaving a gap in knowledge regarding the transient dynamics. To address this gap, this paper explores the evolution of bias in a teacher-student setup modeling different data sub-populations with a Gaussian-mixture model. We provide an analytical description of the stochastic gradient descent dynamics of a linear classifier in this setting, which we prove to be exact in high dimension. Notably, our analysis reveals how different properties... [Read Article]
Explore other publications here.